共计 5073 个字符,预计需要花费 13 分钟才能阅读完成。
导读 | 这篇文章主要介绍了 Pytorch 实现 WGAN 用于动漫头像生成,文中通过示例代码介绍的非常详细,对大家的学习或者工作具有一定的参考学习价值,需要的朋友们下面随着小编来一起学习学习吧 |
WGAN 与 GAN 的不同
去除 sigmoid
使用具有动量的优化方法,比如使用 RMSProp
要对 Discriminator 的权重做修整限制以确保 lipschitz 连续约
WGAN 实战卷积生成动漫头像
import torch
import torch.nn as nn
import torchvision.transforms as transforms
from torch.utils.data import DataLoader
from torchvision.utils import save_image
import os
from anime_face_generator.dataset import ImageDataset
batch_size = 32
num_epoch = 100
z_dimension = 100
dir_path = './wgan_img'
# 创建文件夹
if not os.path.exists(dir_path):
os.mkdir(dir_path)
def to_img(x):
"""因为我们在生成器里面用了 tanh"""
out = 0.5 * (x + 1)
return out
dataset = ImageDataset()
dataloader = DataLoader(dataset, batch_size=32, shuffle=False)
class Generator(nn.Module):
def __init__(self):
super().__init__()
self.gen = nn.Sequential(
# 输入是一个 nz 维度的噪声,我们可以认为它是一个 1 *1*nz 的 feature map
nn.ConvTranspose2d(100, 512, 4, 1, 0, bias=False),
nn.BatchNorm2d(512),
nn.ReLU(True),
# 上一步的输出形状:(512) x 4 x 4
nn.ConvTranspose2d(512, 256, 4, 2, 1, bias=False),
nn.BatchNorm2d(256),
nn.ReLU(True),
# 上一步的输出形状:(256) x 8 x 8
nn.ConvTranspose2d(256, 128, 4, 2, 1, bias=False),
nn.BatchNorm2d(128),
nn.ReLU(True),
# 上一步的输出形状:(256) x 16 x 16
nn.ConvTranspose2d(128, 64, 4, 2, 1, bias=False),
nn.BatchNorm2d(64),
nn.ReLU(True),
# 上一步的输出形状:(256) x 32 x 32
nn.ConvTranspose2d(64, 3, 5, 3, 1, bias=False),
nn.Tanh() # 输出范围 -1~1 故而采用 Tanh
# nn.Sigmoid()
# 输出形状:3 x 96 x 96
)
def forward(self, x):
x = self.gen(x)
return x
def weight_init(m):
# weight_initialization: important for wgan
class_name = m.__class__.__name__
if class_name.find('Conv') != -1:
m.weight.data.normal_(0, 0.02)
elif class_name.find('Norm') != -1:
m.weight.data.normal_(1.0, 0.02)
class Discriminator(nn.Module):
def __init__(self):
super().__init__()
self.dis = nn.Sequential(nn.Conv2d(3, 64, 5, 3, 1, bias=False),
nn.LeakyReLU(0.2, inplace=True),
# 输出 (64) x 32 x 32
nn.Conv2d(64, 128, 4, 2, 1, bias=False),
nn.BatchNorm2d(128),
nn.LeakyReLU(0.2, inplace=True),
# 输出 (128) x 16 x 16
nn.Conv2d(128, 256, 4, 2, 1, bias=False),
nn.BatchNorm2d(256),
nn.LeakyReLU(0.2, inplace=True),
# 输出 (256) x 8 x 8
nn.Conv2d(256, 512, 4, 2, 1, bias=False),
nn.BatchNorm2d(512),
nn.LeakyReLU(0.2, inplace=True),
# 输出 (512) x 4 x 4
nn.Conv2d(512, 1, 4, 1, 0, bias=False),
nn.Flatten(),
# nn.Sigmoid() # 输出一个数 ( 概率)
)
def forward(self, x):
x = self.dis(x)
return x
def weight_init(m):
# weight_initialization: important for wgan
class_name = m.__class__.__name__
if class_name.find('Conv') != -1:
m.weight.data.normal_(0, 0.02)
elif class_name.find('Norm') != -1:
m.weight.data.normal_(1.0, 0.02)
def save(model, filename="model.pt", out_dir="out/"):
if model is not None:
if not os.path.exists(out_dir):
os.mkdir(out_dir)
torch.save({'model': model.state_dict()}, out_dir + filename)
else:
print("[ERROR]:Please build a model!!!")
import QuickModelBuilder as builder
if __name__ == '__main__':
one = torch.FloatTensor([1]).cuda()
mone = -1 * one
is_print = True
# 创建对象
D = Discriminator()
G = Generator()
D.weight_init()
G.weight_init()
if torch.cuda.is_available():
D = D.cuda()
G = G.cuda()
lr = 2e-4
d_optimizer = torch.optim.RMSprop(D.parameters(), lr=lr, )
g_optimizer = torch.optim.RMSprop(G.parameters(), lr=lr, )
d_scheduler = torch.optim.lr_scheduler.ExponentialLR(d_optimizer, gamma=0.99)
g_scheduler = torch.optim.lr_scheduler.ExponentialLR(g_optimizer, gamma=0.99)
fake_img = None
# ########################## 进入训练 ## 判别器的判断过程#####################
for epoch in range(num_epoch): # 进行多个 epoch 的训练
pbar = builder.MyTqdm(epoch=epoch, maxval=len(dataloader))
for i, img in enumerate(dataloader):
num_img = img.size(0)
real_img = img.cuda() # 将 tensor 变成 Variable 放入计算图中
# 这里的优化器是 D 的优化器
for param in D.parameters():
param.requires_grad = True
# ######## 判别器训练 train#####################
# 分为两部分:1、真的图像判别为真;2、假的图像判别为假
# 计算真实图片的损失
d_optimizer.zero_grad() # 在反向传播之前,先将梯度归 0
real_out = D(real_img) # 将真实图片放入判别器中
d_loss_real = real_out.mean(0).view(1)
d_loss_real.backward(one)
# 计算生成图片的损失
z = torch.randn(num_img, z_dimension).cuda() # 随机生成一些噪声
z = z.reshape(num_img, z_dimension, 1, 1)
fake_img = G(z).detach() # 随机噪声放入生成网络中,生成一张假的图片。# 避免梯度传到 G,因为 G 不用更新, detach 分离
fake_out = D(fake_img) # 判别器判断假的图片,d_loss_fake = fake_out.mean(0).view(1)
d_loss_fake.backward(mone)
d_loss = d_loss_fake - d_loss_real
d_optimizer.step() # 更新参数
# 每次更新判别器的参数之后把它们的绝对值截断到不超过一个固定常数 c =0.01
for parm in D.parameters():
parm.data.clamp_(-0.01, 0.01)
# ================== 训练生成器 ============================
# ############################### 生成网络的训练 ###############################
for param in D.parameters():
param.requires_grad = False
# 这里的优化器是 G 的优化器,所以不需要冻结 D 的梯度,因为不是 D 的优化器,不会更新 D
g_optimizer.zero_grad() # 梯度归 0
z = torch.randn(num_img, z_dimension).cuda()
z = z.reshape(num_img, z_dimension, 1, 1)
fake_img = G(z) # 随机噪声输入到生成器中,得到一副假的图片
output = D(fake_img) # 经过判别器得到的结果
# g_loss = criterion(output, real_label) # 得到的假的图片与真实的图片的 label 的 loss
g_loss = torch.mean(output).view(1)
# bp and optimize
g_loss.backward(one) # 进行反向传播
g_optimizer.step() # .step() 一般用在反向传播后面, 用于更新生成网络的参数
# 打印中间的损失
pbar.set_right_info(d_loss=d_loss.data.item(),
g_loss=g_loss.data.item(),
real_scores=real_out.data.mean().item(),
fake_scores=fake_out.data.mean().item(),
)
pbar.update()
try:
fake_images = to_img(fake_img.cpu())
save_image(fake_images, dir_path + '/fake_images-{}.png'.format(epoch + 1))
except:
pass
if is_print:
is_print = False
real_images = to_img(real_img.cpu())
save_image(real_images, dir_path + '/real_images.png')
pbar.finish()
d_scheduler.step()
g_scheduler.step()
save(D, "wgan_D.pt")
save(G, "wgan_G.pt")
到此这篇关于 Pytorch 实现 WGAN 用于动漫头像生成的文章就介绍到这了。
正文完
星哥玩云-微信公众号
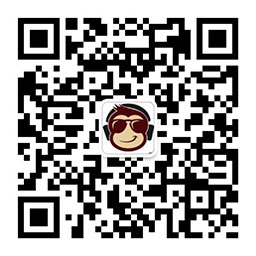